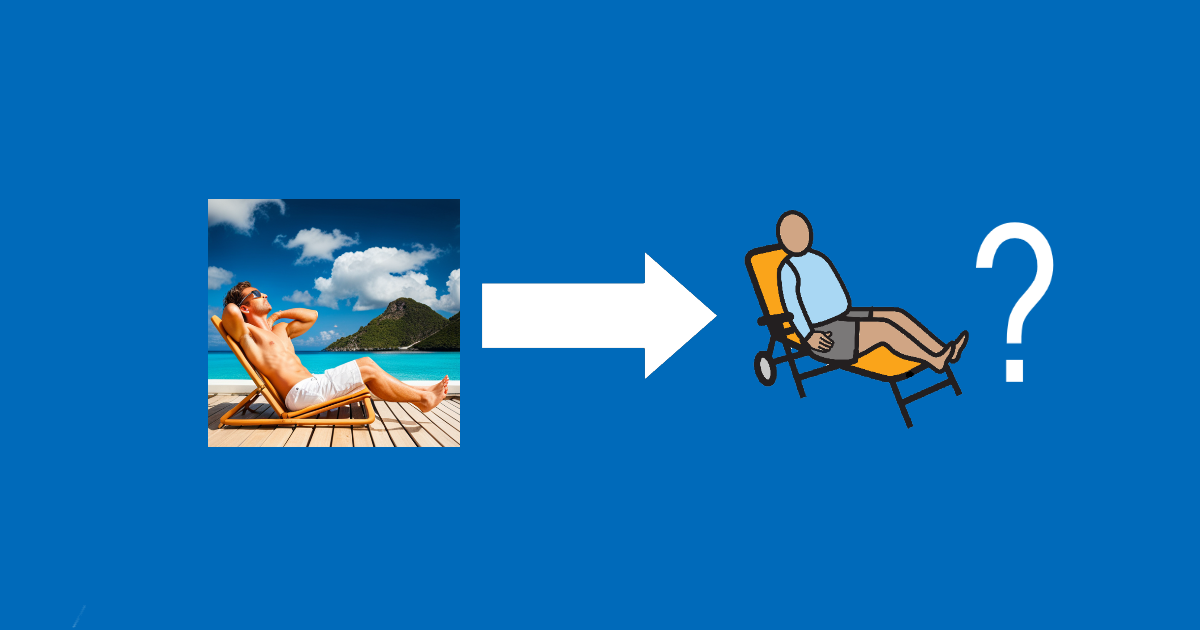
E.A. Draffan
Stepping into the world of AI, Icon Recognition, Human Perception and AAC Symbols.
Over the last couple of months we have been researching how inconsistency can hamper automated image recognition after pre-processing and feature abstraction, but the advent of Stable Diffusion as a deep learning model allows us to include visual image text descriptions alongside image to image recognition processes to support our ideas of symbol to symbol recognition and creation.
Stable Diffusion - "The input image on the left can produce several new images (on the right). This new model can be used for structure-preserving image-to-image and shape-conditional image synthesis." https://stability.ai/blog/stable-diffusion-v2-release
Furthermore, with the help of Professor Irene Reppa and her project team researching “The Development of an Accessible, Diverse and Inclusive Digital Visual Language” we have discovered many overlaps in the work they are doing with icon standardisation. Working together we may be able to adapt our original voting criteria to provide a more granular approach to ensuring automatically generated AAC symbols in the style of a particular symbol set allow for ‘guessability’ (transparency) and ease of learning whilst also making them appealing based on a much more inclusive set of criteria. The latter have been used by many more evaluators over the last 8 years as is mentioned in this blog “When the going gets tough the beautiful get going.”
One important finding from Professors Reppa's previous research was that when “icons were complex, abstract, or unfamiliar, there was a clear advantage for the more aesthetically appealing targets. By contrast, when the icons were visually simple, concrete, or familiar, aesthetic appeal no longer mattered.” The research team are now looking at yet more attributes, such as consistency, complexity and abstractness, to illustrate why and how the visual perception of icons changes within groups and in different situations or environments.
In the past we have used a simple voting system with five criteria using a Likert scale with an option to comment on the symbol and the evaluators have been experienced AAC users or those working in the field (which is small in number). On previous symbol survey occasions it has usually been the individual evaluator’s perception of the symbol, as seen in a text comment, that provided the best information. But, the comments have been small in number and the cohorts not necessarily representative of a wider population of communicators.
There is no doubt in my mind that we need to keep exploring ways to enhance our evaluation techniques by learning more from icon-based research, whilst being aware of the different needs of AAC users, where symbols may have a more abstract representation of a concept. This process may also help us to better categorise our symbols in the Global Symbols repository to aid text based and visual searches for those developing paper-based communication charts, boards and books as well as linking to the repository through AAC apps such as PiCom and Cboard.